We are stepping through the five common myths that are keeping you from taking full advantage of AI. In the first article, we discussed getting the basics right. Today, we’re jumping into myth #2, which is…
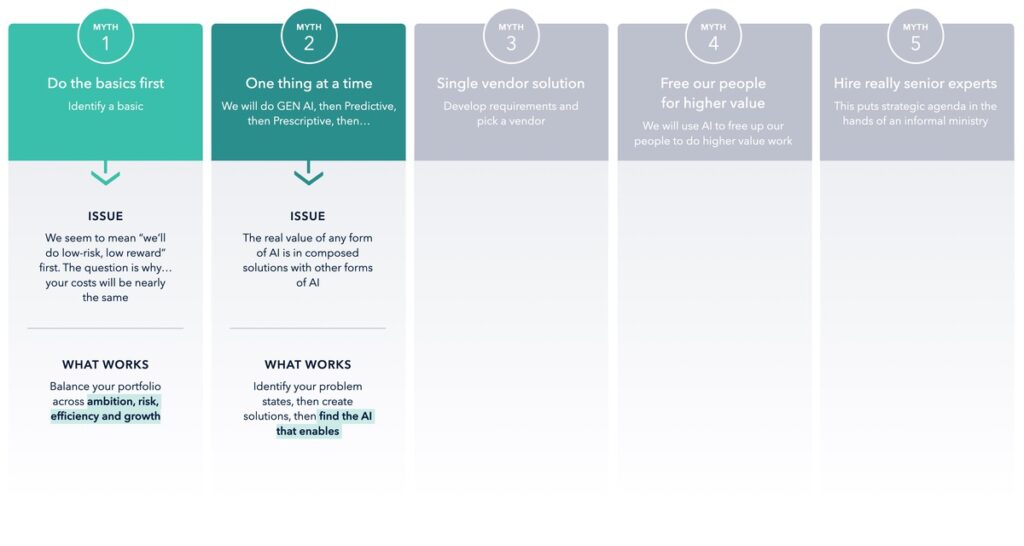
One Thing At A Time
This myth is a close cousin of our first myth… We Gotta Get The Basics Right. It’s the reasonable but utterly faulty assertion that an organization should implement one form of AI at a time. Everyone’s spun up about Generative AI (although we are blessedly starting to move off the peak of the hype cycle on Gen AI), so they’re scrambling to put their Gen AI platform in place. Or…an organization wants to start inflicting dynamic bots on its customers, so it is embarking on a massive exercise to put in an enterprise Conversational AI platform. Or, you really want to pump up your analytics by getting a robust predictive AI platform in place.
None of these are bad things to do. However, treating these as separate and unrelated initiatives guarantees a sub-optimal outcome. Or, to take it out of consultant-speak, you ain’t doin’ it right.
Like all things digital, AI delivers more valuable solutions when it is a composite or composed solution. Like playing with Legos, you want to snap digital capabilities together to create a more valuable digital offering. In the world of AI, that means a composite AI solution where you snap together, say, Generative with Conversational. Those are closely related things anyway – technically, conversational is a subset of generative, but at the useful and deployable level at present, you will almost certainly have separate platforms for those two things. Snapping Predictive AI together with both Generative and Conversational creates even greater value.
The question is how you avoid a siloed approach to AI. The answer, as it will be with most of our myths, is to begin with the problem statement.
A use case-based approach is all the rage right now, but we urge you to take a step back and identify the problem statement(s) that is/are most vexing and/or most valuable to solve. If the problem statement is robust, use cases will fall out quickly, easily, and with greater effectiveness.
Following the identification of problem statements and attendant use cases, it is quick work to develop comprehensive use cases that allow for detailed requirements to emerge. At that point, you will be able to identify enterprise-wide requirements across, in our example above, Generative, Conversational, and Predictive platforms… Almost certainly including a Prescriptive AI add-on, but I digress. If you aren’t aware of Prescriptive AI and how it makes Predictive or any other form of discriminative AI more valuable, give us a shout.
As a wrap up – take a problem statement-based approach to identifying AI uses and requirements, and you will develop a more comprehensive, valuable, efficient, and effective AI portfolio.
Myth #3 – There’s a Single Vendor Solution is up next. Also, we’re a step closer to the one that no one expects…Myth #4. But we aren’t telling what it is just yet.
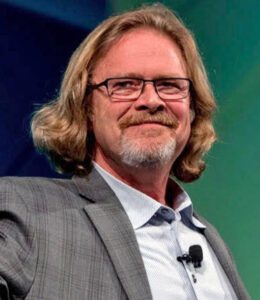
Rik Reppe
leads the Cortico-X Innovation Practice, where he oversees efforts to disrupt the status quo and drive transformative change by breaking traditional molds to envision and create the future.